Fast Simon’s Deeply Personalized Search & Recommendation Engine
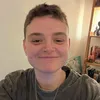
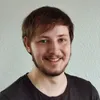
Published September 15, 2024.

Fast Simon’s advanced personalized solution enhances any ecommerce experience with ease and speed, creating the ideal conditions for optimized customer satisfaction and conversions.
Our platform offers intricately tailored recommendations, ready customization tools, white glove service and seamless integrations with existing tech stacks. With a formula that elevates the ecommerce experience for both the merchant and client, our AI is powering forward with models of the future.
Privacy First
As we look towards a cookie-free future, maintaining acceptable privacy standards is essential, and Fast Simon’s on-site behavior personalization model meets this requirement. As we are able to ensure a secure customer experience and a positive execution, the merchant needs only to focus on strategy whilst we take care of the rest.
Upcoming changes to tougher consumer privacy laws will not be an issue for Fast Simon’s personalization methods, as they do not impede on personal privacy. Personal data is not required in order to create effective campaigns, the advanced algorithm is capable of creating accurate recommendations from on-site actions alone.
Recommendations
Fast Simon’s module integrates easily into existing infrastructure and software, without requiring API software updates. Tailored recommendations are implemented effortlessly via onsite actions, ensuring the customers privacy is always protected.
We model user interactions to train our recommendation services, in the following formats:
User-to-Items recommendations: best items to match user based on site search queries, product views, purchases and other behavioral signals
Item-to-Items recommendations: API fetching similar products from a selected catalog product. This is our primary model user interaction. Our AI relies on product attributes, product text, product images, and shoppers’ big data (for viewed together, carted together, purchased together) to create these recommendations. We also use visually based item-to-item recommendation
Session-based recommendations: anonymous interactions gather session time data to enhance ongoing interactions
Our primary user interaction model is Item-to-Item recommendations. These use the following engines to function:
- Product attributes. These are extracted from the product catalog, then a feature representation of each product is created according to its attributes, then the similarity between each of the feature representations is computed. Merchants are able to control which attributes are relevant so they are taken into account in this process.
- Product text: natural language processing is used to extract meaningful keywords from product text (title and description), then representations are created based on these keywords which are used to source similar items.
- Visual similarity: image embeddings for each product are created, then we compute the similarity between products based on image representations
- Behavioral similarity: shoppers behavior metric is computed based on shoppers’ events such as product views, add to cart and purchases. If two products are viewed in one session, the products are classified as related. Sourcing based on “wisdom of the crowds”
Real-time personalized filters: filters specific to previous queries and interactions
We use an implicit feedback model to gather information based on interactions, using sources such as clicks, scrolls and page views; machine learning based on consumer interaction with the application. We also use the items catalog visual and textual data to enrich the information the algorithm is able to gather for recommendations.
Machine Learning Embedding
Embedding looks set to be the future of tech, as transforming raw data into representations intuitively is difficult. Feature engineering for relational and human behavior data is not only time consuming, but also a challenging process. Embedding provides an appealing alternative by reducing the dimensionality of categorical and continuous variables. After discerning which clusters, actions and classifications are significant, this data can be turned into profiles.
Fast Simon builds on this technology regarding images, reducing the time and effort otherwise needed to perceive trends in behavioral patterns. Our Visual Similarity functionality aids users in identifying products without requiring textual queries. Since it is based on subjective judgment and is domain specific, Visual Similarity is highly ambiguous.
We use deep convolutional networks to create product image embeddings based on catalog images. The embeddings representations of the visual components of the product. The networks are trained to create embeddings that closely resemble those of similar products, and differ from others. This allows us to quickly calculate the distance between user images and product images, and also between different catalog products. This allows us to recommend and promote visually similar products that best match the user’s preferences and personal interests.
These embeddings are used in different stages of the customer journey- visual search, lookalike recommendations and personalization based on visual similarities. This technique is also employed in product page recommendations, with the merchant option to display upsell or cross-sell potentials. This is enhanced through text classifiers based on product title, description and attributes. ML algorithms are used to train these classifiers with domain-based vocabulary.
Integration
Our enhanced API is easily integrated to any platform, to ingest data or deploy recommendations. This creates a convertible, customizable solution that was designed in order to be able to scale with your business as it grows. Working with existing tech stacks, the power of our platform can be accessed immediately.
Our advanced algorithms don’t rely on guesswork, and for anything unclear our team is on hand to provide personalized solutions that retain privacy and optimize user experience.
Fast Simon’s API is built with designers in mind, so easily integrated into business via leading web clients, and readily translated into other languages. This superior tech stack integration is easy and advantageous.
Every-Page Personalization
The evolution of standard search engine optimization points towards every-page website personalization. Through filter recommendations and widgets, Fast Simon provides this, offering customers the uniquely tailored content they require. Machine learning knowledge returns most relevant items for users through search, filters and recommendations within milliseconds. The API transforms customer queries into conversions, providing a fully personalized and powerful consumer journey.
Each page displays optimized placement of personalized recommendations in terms of user experience. For example, cross-sell and upsell products are prominently placed on the Cart and Checkout pages, as well as product pages. Similarly the homepage displays personalized recommendations based on past interest and micro-segmentation.
Optimization for Anonymous Users
The ‘Cold Start’ problem is the issue surrounding providing anonymous or first time users satisfactorily personalized experiences, leading them to turn them into converters. Since first time users constitute a significant part of the audience, their engagement is meaningful, predicting their wants and needs essential.
Fast Simon solves this problem through session based data leading to increased first-time sales. This is done via machine learning algorithms and content extraction. As soon as the customer begins their online experience data is collected to start offering personalized recommendations and personalized search and browsing results..
The second part to the ‘Cold Start’ problem relates to just-added products with no relevant interactions to-date. Our extraction algorithm solves this problem by automatically extracting text and information from photos, product titles and descriptions to educate the AI on similar and related products in real time. This means that it is not necessary for products to have previous interactions for them to be recommended.
Our API enables connections with existing on-site products to be forged within minutes of adding the product. Intricate details (personal or categorical) are no longer required to engage customers and find them their desired products.
Optimized Cross-sell and Upsell
Using AI machine learning data configuration, Fast Simon’s algorithm decodes the enigma within images to recommend similar or related items, increasing sales.
Item-to-item relationships can be monitored, and we are able to extract information from items descriptive text with natural language processing. With our Visual Discovery module and Hyper-tagging we utilize convolutional neural networks to create associations with other products.
Recommendation merchandising is offered, allowing the merchant to restrict input recommended products from the desired category to be from a specific category they choose. This allows recommendations to be tailored to the merchants needs.
When discerning item-to-item similarly, merchants can configure specific relevant product attributes for summoning similar products, in accordance with business models and rules. These offered selected attributes are site-specific originating from each store’s catalog.
Using implicit feedback data Fast Simon provides quick and profitable Cross-sell and Upsell recommendations with high conversion rate results.
Fast Simon also offers Shop-The-Look recommendations, decomposing an image into identifiable products. We apply object detection deep networks to identify specific items and their bounding boxes and contours. Knowing where each item is located on the image enables us to use deep convolutional networks to build embeddings for the specific parts of the image, then apply visual similarity to those parts alone.
Personalized Emails, SMS & Facebook Ads
Email and SMS marketing is a powerful tool forging lasting bonds between customers and merchants. Cross-sell and Upsell emails are especially fruitful, and a key to the success of these emails is available data. Fast Simon is able to leverage onsite behavior to create data required for successful emails using AI machine learning recommendation technology, used to trigger intent-based flows showing customers highly personalized relevant products.
Fast Simon’s easy integration with email and SMS marketing platforms such as Klaviyo is successful as a result of the precise attention to detail that outstanding email marketing needs.
Fast Simon’s integration with Facebook uses gathered eCommerce data to inform Faceboook’s advert targeting segments. This shared data will ensure that highly relevant personalized ads will reach the relevant specific audience, as it sends traffic data that has proven to produce conversions.
Looking Forward
These are some of the personalization technologies that the Fast Simon solution employs today, but we are always building and growing. Our roadmap involves the creation of user profiles without infringing data privacy requirements and a closer model of machine learning embedding, using data through deep collaborative filtering. As the ecommerce world moves into a more highly personalized future, Fast Simon moves right along with it.